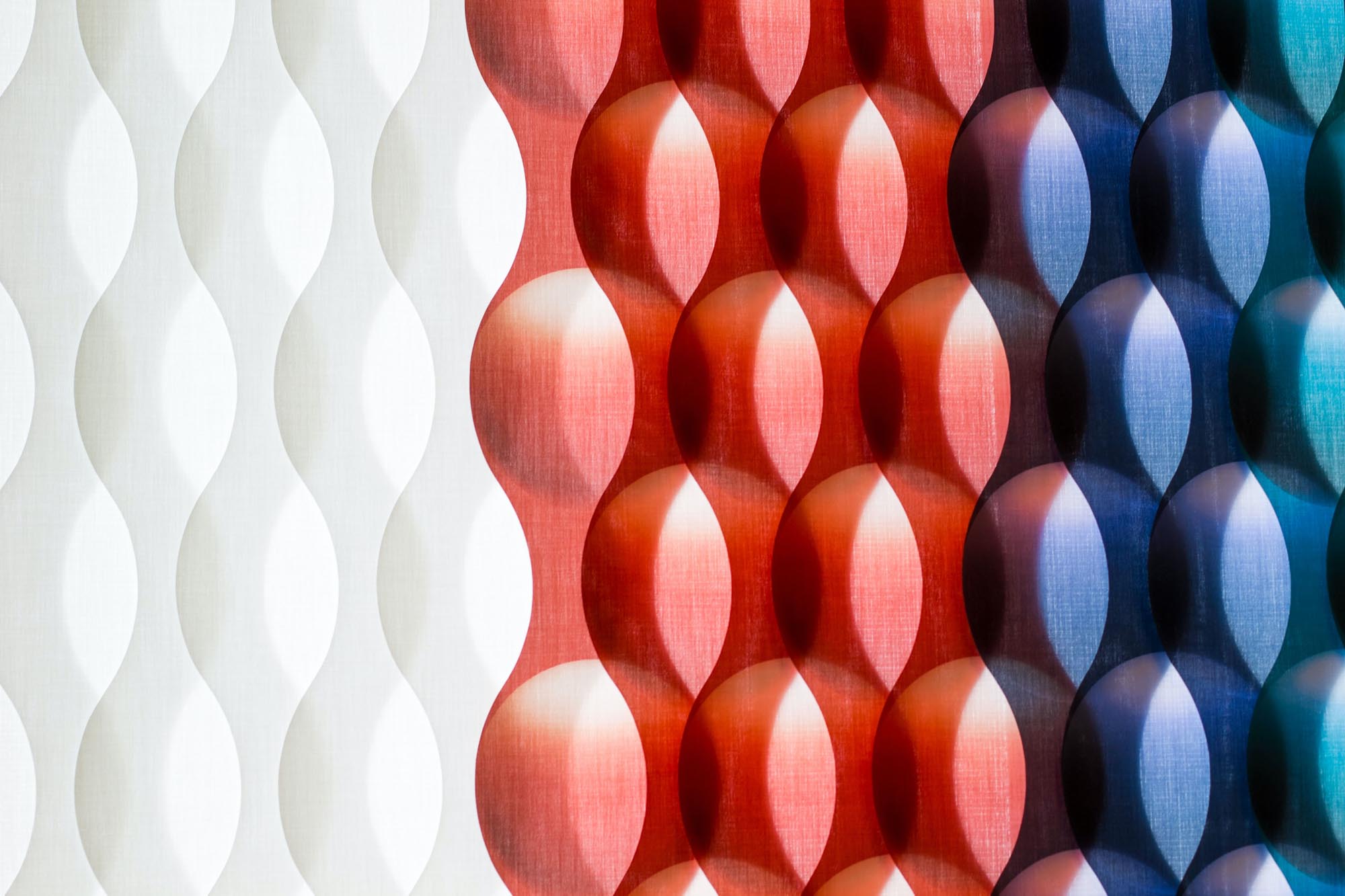
The NHS has started trials of a machine learning (ML) model that will help predict the demand for beds and ventilators as hospitals struggle with resources in the fight against COVID-19.
Developed by researchers at the University of Cambridge, the ‘Capacity Planning and Analysis System’ (CPAS) has this week been deployed in alpha trials at four UK hospitals.
Using depersonalised data — collected by Public Health England’s COVID-19 ‘Hospitalization in England Surveillance System’ — the ML system aims to predict how many ICU beds and ventilators will be required a week in advance by forecasting patient intensive care needs and overall recovery times.
NHS Digital Chief Medical Officer Professor Jonathan Benger commented that: “CPAS allows individual hospitals to plan ahead, ensuring they can give the best care to every patient. At the same time, the wider NHS can ensure that the ventilators, other equipment and drugs that each intensive care unit will need are in place at exactly the time they are required.”
It is hoped that the systems can provide a robust demographic picture of hospital patients during the crisis, detailing medical conditions and how that compares to specific hospitals on local and national scales.
While one of its main goals is forecasting resource requirements, it is thought the system could also be used as a ‘simulation environment’ in which researchers can run treatment scenarios such as rapid bed increases or grouping/separation of patients with particular medical profiles.
Benger noted that: “In the longer term, it is hoped that CPAS can be used to predict length of hospital stay, discharge planning and wider intensive care demand in the time that will come after the pandemic.”
Cambridge Adjutorium
The trial is the result of a partnership between NHS Digital and the University of Cambridge. The CPAS system has been built using the machine learning engine Cambridge Adjutorium.
Cambridge Adjutorium was originality developed by Professor Mihaela van der Schaar and John Plummer Professors as a prognostication tool in the treatment of cardiovascular disease. Since then it has been expanded for use in the treatment of an array of diseases and conditions such as breast cancer and cystic fibrosis.
The machine learning model they have developed for COVID-19 predicts patients’ death, discharge and ICU admissions probabilities in a timeline ‘horizon’ of 14 days.
In an internal technical document, the researchers note that: “For each time horizon T, these data sets are created by including all patients who have follow up for T days, and then binary outcomes are created as follows: If the patient experienced the outcome by time T, a label 1 is attached to the patient, otherwise a label 0 is attached. The process is then repeated for all time horizons in { 1 day, . . . , 14 days }. After obtaining the probability curve over all the 14-time horizons, the final probability curve is smoothed via a piece-wise monotonic interpolation.”
“To capture uncertainty, we repeat the process above N times (using bootstrapped subsets of the data set) to obtain N versions of the model. The final prediction is obtained by averaging the predictions made by the N models.”

Privacy
Privacy and health data is a topical subject, with National Data Guardian for Health and Social Care Fiona Caldicott last week stressing concern that with the growing demand for access to health data, an open public discussion should be conducted to better understand the public’s view on the use of NHS data.
Read More: Healthcare Data Sharing: “Public Dialogue” Needed, as Consultation Planned
However, with regards to the data collected by Public Health England’s COVID-19 ‘Hospitalization in England Surveillance System’ it has all been depersonalized before it is ingested into the Cambridge Adjutorium system.
Professor van der Schaar commented that: “Although the system uses data from individuals to build its models, the system does not make treatment decisions about individual patients. Rather, by aggregating that data we can make more accurate predictions about larger groups, at the level of a hospital, a trust, a region or nationally. So while we can say with a high level of confidence that 30 out of 40 ITU beds in a hospital will be occupied next week, we are not trying to predict which patients will be in them.”