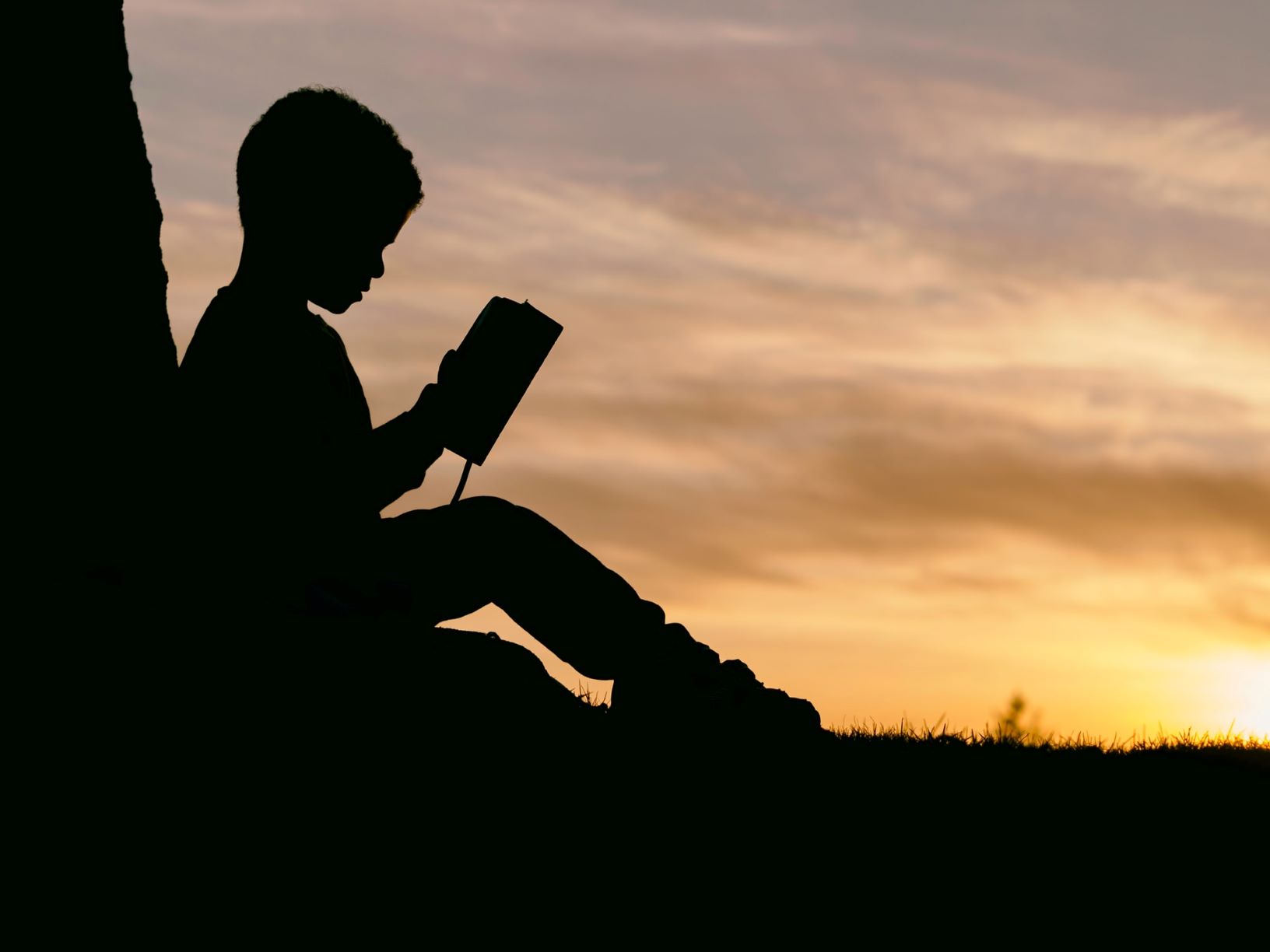
BCS spent two years working with the Office for Artificial Intelligence and the Office for Students supporting their work to scale the education pipeline of data scientists and AI practitioners at Masters level to meet the needs of industry and society, writes Dr Bill Mitchell, director of policy at the BCS – The Chartered Institute for IT.
For employers keen to ensure they have the right AI competencies to be future proof as AI becomes ubiquitous in the workplace: firstly, they must understand its limitations. Despite the ludicrous hype about AI (both positive and negative) it is genuinely transformative, but in a highly constrained way.
Companies need the right competencies to navigate through the snake-oil to successfully adopt AI products and services that will help their businesses be resilient in the face of COVID-19 and grow in the face of future economic shocks, rather than dissipating precious time and energy on shiny new AI tech that over promises and under delivers.
The consistent message we got from employers about competencies is the urgent need to develop diverse interdisciplinary teams that are highly skilled at ethically:
- transferring a deep knowledge of data science and artificial intelligence into business contexts
- engineering AI systems that meet business needs
- managing the adoption of AI technologies and maximising their value across strategic business units.
Why has that got anything to do with scaling up an ethical MSc pipeline?
Table 1 shows the ONS data on the proportion of jobs that are likely to be automated over the long term based on the level of education required for those jobs. It shows that 87% of jobs requiring a degree are at a low risk of automation, compared with 98.8% of jobs that require an A-level or less that are at high risk of being automated. AI is maturing at a remarkable rate in a range of high-value niche areas, as illustrated by a recent article in the Guardian written by a machine learning algorithm. That article wasn’t written from scratch by the machine learning algorithm, it had human oversight and guidance, but it shows how mature and sophisticated AI has already become. We’re not quite yet at the stage where the AI-bots are completely taking over, but we are very soon going to be at the point where jobs are going to be at the very least dramatically augmented by AI.
Table 1: ONS data on proportion of main jobs at long-term risk of automation by level of education
Qualification | Lower than GCSE | A Level or GCSE | Higher education | Degree |
Low risk | 0.2% | 5.3% | 7.5% | 87.0% |
Medium risk | 13.6% | 57.5% | 9.9% | 18.9% |
High risk | 39.0% | 59.8% | 1.2% | 0.0% |
Let’s look at the ONS data from the point of view of competitive market forces, particularly the need to be resilient, improve productivity and maintain growth in future pandemics and economic factors such as business outside the EU. It suggests employers will need to attract, retain and develop graduates with the right data science and AI skills to fill high-value non-automatable jobs, whilst making the best possible use of those skilled graduates to manage the automation of jobs with lower educational requirements as fast as they can. For that to happen means recruiting graduates with sophisticated data science and AI skills. Until AI functions have become thoroughly commoditised these graduates will probably need to be at Masters level. Hence, meeting the employer needs for developing interdisciplinary teams mentioned above means engaging with suitable MSc programmes as a source of recruitment.
At the start of the article we talked about AI being transformative in particular niche areas, so what are those? An excellent accessible introduction to these, and the report we based our advice to the Office for AI on, is the Royal Society report “Machine learning: the power and promise of computers that learn by example”. That report really drives home the need for companies to be data literate and statistically literate as a starting point if they are going to get value from AI. Fundamental to developing that type of literacy is a solid understanding of the scientific method of experimentation and investigation as it applies within a business context. We described the business contextualised scientific method in our report on MSc graduate skills to the Office for Students as follows. Employees managing the adoption of AI systems should have extensive knowledge and understanding of the principles, concepts and techniques for:
- systematically identifying all relevant data to a problem domain in an organisation,
- formulating a comprehensive range of plausible hypotheses based on rigorous exploration and experimentation with the data,
- the iterative evaluation and modification of hypotheses to develop those of optimal utility to the decision-making process.
At a superficial level that sounds fairly straightforward. A little thought suggests otherwise. When is data relevant to a problem domain and when isn’t it? How do we find data in the first place, and how does one know when you’ve got a complete set of data? What do you do if new data comes along that you didn’t know about? How do you gather the data from the various sources and harmonise them within suitable data structures? How do you synthesis hypotheses, how do you evaluate them, how exactly do you modify them to get something better?
Note all this is nothing to do with AI per se, it’s what we should think of as an essential scientific aspect of business intelligence. If companies can’t understand and adopt the scientific method as part of their data and AI strategies they’ll be swimming in AI snake-oil before the next pandemic hits.
Another really important point to highlight from our conversations with employers is the need for ethically adopting and using AI products and services. That turns out to be harder than you think. That’s because AI is stunningly brilliant at throwing out unintended consequences. AI is superb at exposing gaps in data governance that no one in an organisation had thought about. Adopting AI in a truly transformative way that is ethical is a result of organisations working collaboratively across silos, with shared openness, responsibility and accountability. That never goes wrong does it?
Work BCS did with the government’s independent Centre for Data Ethics and Innovation identified the following list that should trigger putting in place robust ethical data governance processes. AI systems needing the highest possible level of ethical governance are ones:
- that are automated systems that must process data streams in real-time
- that use probabilistic self-learning algorithms to inform decisions that will have significant consequences for people
- where it is difficult to uncover how decisions are derived
- where contestability of decisions is not deterministic and
- ultimately decisions rely on best judgment that requires understanding of the broader context.
In summary, companies have a good shot of successfully adopting AI if they have diverse interdisciplinary teams who can ethically handle the science, the engineering and the management of AI products and services. Now is a good time to find a university near you running one of the new MSc data science or AI programmes the Office for Students have commissioned to meet the needs of industry.