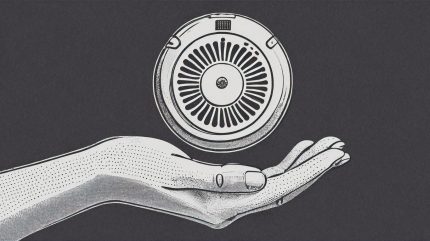
If 2024 was the year of the large language model (LLM), then 2025 will be the year we collectively think about downsizing. That, at any rate, is a prevailing view among analysts in the Gen AI space, many of whom believe that small language models (SLMs) are about to hit the mainstream.
While they may not have the scale and complexity of an LLM – some of which boast parameters in the trillions – SLMs are cheaper, more efficient, faster to train and easier to deploy. Ranging from a few million to a few billion parameters, they are essentially the same as any other gen AI neural network model, except for their relatively small size.
SLMs are designed to be used by enterprises for smaller, specialised tasks that don’t require huge datasets. These might include customer support chatbots specific to a given product or models for analysing market trends. They’re also useful in cases where internal data is sensitive and needs to stay inside the company – for instance in the financial or healthcare sector.
“They are designed for resource-constrained environments, and suited for leveraging on devices that cannot accommodate large models,” notes Mohan Varthakavi, VP for AI and Edge at Couchbase. “For instance, they are essential for enabling AI on mobile and edge devices.”
Since November 2022, when OpenAI launched ChatGPT, enterprises and consumers alike have been in thrall to the promise of LLMs. Tech companies have developed bigger and better models. Businesses, meanwhile, have used these tools to improve customer service, better manage workflows, and augment human expertise. According to the International Data Corporation, worldwide enterprise spending on Gen AI solutions is expected to grow from $16bn in 2023 to $150bn by 2027.
As such, LLMs are unlikely to lose their lustre any time soon. As Ray Valdes, research VP analyst at Gartner, points out, “the percentage of SLM-based solutions in the enterprise is still vastly outweighed by conventional large models from commercial cloud vendors.”
That said, we are seeing a widespread acknowledgement of their downsides – and a newfound openness to other options. In recent months, Microsoft, Meta, Google and French startup Mistral have all released SLMs with just a few billion parameters. We are also seeing the launch of AI PCs, computers with inbuilt neural processing units perfect for running your own nifty small language model.
“Recently it seems that foundation models have reached a point of diminishing returns in terms of scaling model sizes,” remarks Valdes. “In the past, increasing the number of parameters by 10x from GPT3 to GPT4 resulted in a very powerful and effective model. AI researchers no longer believe this development strategy will hold.”
The case for SLMs
As the thinking goes, LLMs may be the best choice for complex or open-ended tasks. But they’re also expensive – at times prohibitively expensive – and can be hard to monitor and maintain.
On top of that, training these models requires a vast amount of computational power, with a carbon footprint to match. Developing GPT-3, which has 175bn parameters, produced an estimated 552 tons of CO2. That’s comparable to the annual emissions for more than a hundred cars.
“Meta’s largest model, Llama 3.1, is 405 billion parameters,” says Bradford Levy, an assistant professor of accounting at the University of Chicago Booth School of Business. “It took more than 50 days training on 16,000 graphics processing units (GPUs) to train that model, which is a huge amount of compute. If a GPU fails, it can slow down or completely halt the entire training process. That’s something you don’t have to think about at all when you’re training a model across eight or 24 GPUs.”
There can be data security issues too. Many businesses, worried about privacy, are loath to use a vendor’s cloud data centre, but simply don’t have the resources necessary to deploy an LLM on site. An SLM could be easily hosted on premises, assuaging their concerns.
Then there’s the problem of hallucinations (false outputs and unsubstantiated answers), which are a common menace with LLMs. “Some research points to the finding that the larger the model, the harder it becomes to manage hallucinations,” remarks Juan Bernabe Moreno, director of IBM Research Europe for the UK and Ireland. “But if the model is smaller, it’s more controlled, more transparent, and easier to trust.”
Limitations and trade-offs
From a performance perspective, there are likely to be certain trade-offs. SLMs may not be able to compete with their larger counterparts in terms of completeness and relevance of the results. “If you compare a small language model with a very large language model, the performance points might be still in favour of the larger one,” notes Moreno.
‘Small’ is a relative term. Today’s iteration of SLMs often have similar parameter numbers to older LLMs. And as recent studies have demonstrated, the best SLMs can actually outperform larger models in specialised tasks.
In one example from the legal field, a tiny SLM (200m parameters) outshone GPT-4 (1.8tn parameters) at identifying unfair terms in user agreements. As Ed Challis, Head of AI Strategy at UiPath, points out, “SLMs are better at handling industry-specific terminology and queries and have lower error rates due to their specialised training.”
Many analysts believe the performance question needs to be weighed against other, equally salient factors. “In a lot of cases, maybe performance increases by 1 or 2% with an LLM, but do customers actually care about that?” argues Levy. “If it costs 10 times as much to host, and the customer satisfaction scores aren’t really that different, then why are you going down that path?”
As a rule, the suitability of a given model will depend upon your chosen use case. If you want to feed your model on vast amounts of data, leading to an all-purpose AI, you’re probably best off sticking with an LLM.
On the other hand, if you’re looking to fulfil a specific business need, an SLM would likely suffice. Just make sure your data is high-quality, warns Adam Lieberman, chief AI officer at Finastra. “If your data sets aren’t robust or comprehensive,” says Lieberman, “then the performance of your model may suffer, which can lead to some inaccurate outputs or maybe even some biased results.”
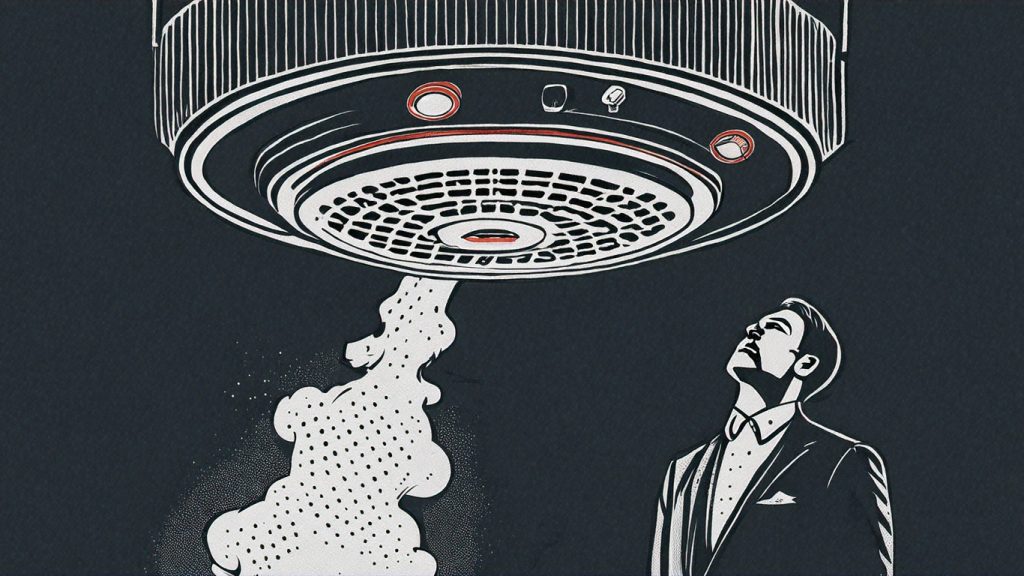
Making sense of the sprawl
SLMs, therefore, are not a silver bullet, and they’re not going to replace enterprise LLMs any time soon. According to Gartner, SLMs are growing at a pace faster than the rest of the market, with their deployment due to double or triple over the next few years. But they will nonetheless remain in the minority, with most businesses deploying these tools only as part of a broader mix.
Varthakavi at Couchbase believes that many enterprises will settle on a hybrid approach. “We will likely see many use cases where LLMs and SLMs are complementing each other – with LLMs providing general foundational data and SLMs providing domain-specific data,” he says.
Valdes at Gartner is less optimistic about how well they’ll work together. In his view, enterprises will adopt many systems of varied origins, leading to what he calls ‘Gen AI sprawl.’ “The result is not pretty but it will constitute reality over the next few years,” he remarks. “What is important over the near term is that organisations ensure they get value and benefit from this disordered and volatile ecosystem.”
Consolidation will come, thinks Valdes, further down the line. In the meantime, businesses could stand to gain from taking a very deliberate approach to generative AI. If the last few years have been characterised by experimentation – playing around with different possibilities and seeing what happens – 2025 may be the year for firming up your strategy. According to Moreno at IBM, SLMs ought to be an integral piece of that.
“They offer the possibility of incorporating your own data in a way that can differentiate your business,” he explains. “The antithesis of that would be companies just using general models because every other company can do the same. But we want to enable AI creators, which means that each and every company can use Gen AI to gain a competitive advantage.”
It really comes down to understanding the business case for any model you pick. Size clearly isn’t everything when it comes to Gen AI. But intentionality just might be.