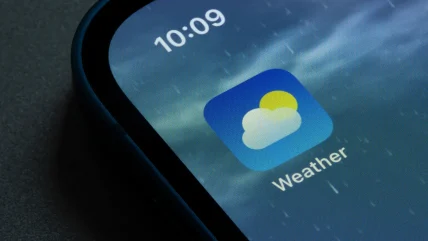
Google DeepMind has introduced a new artificial intelligence (AI) model, GenCast, designed to improve weather forecasting and the prediction of extreme conditions. In a paper published in Nature, the team explained how GenCast delivers weather forecasts with increased accuracy, extending up to 15 days in advance. This development is part of ongoing efforts to enhance weather predictions as climate change results in more frequent and severe weather events.
How GenCast works
In contrast to traditional models that produce a single forecast, GenCast is said to generate an ensemble of over 50 individual predictions, each representing a possible weather scenario. This approach provides a broader view of potential weather developments, helping decision-makers plan for different outcomes. The model uses a diffusion technique, a form of generative AI typically used in other fields like image generation, but adapted for weather forecasting. By processing four decades of historical weather data, including variables such as temperature, wind speed, and pressure, GenCast is claimed to predict future weather conditions based on the most recent data available.
GenCast has been tested against the European Centre for Medium-Range Weather Forecasts’ (ECMWF) ENS system, a widely used operational model, according to the Google DeepMind teams. They also stated that the results showcase GenCast as more accurate than ENS in over 97% of forecast targets, with a 99.8% accuracy rate for forecasts made beyond 36 hours. The model showed improvements in predicting extreme weather events, including heatwaves, cold fronts, and high wind speeds, which are often more difficult to forecast accurately.
Additionally, the new AI model is said to have demonstrated improved forecasting capabilities for tropical cyclones, offering more precise predictions of their tracks. This could help improve the timeliness and accuracy of warnings, which are important for planning evacuations and preparing for storm impacts.
Using a single Google Cloud TPU v5, GenCast is able to generate a complete 15-day forecast in approximately eight minutes. In comparison, traditional ensemble forecasting models, such as those from ECMWF, typically require more time and computational resources. This increased speed could offer advantages for real-time decision-making and improve the efficiency of planning and response efforts.
GenCast’s improved forecasting also has potential applications in other sectors, such as renewable energy. A trial suggested that GenCast was more accurate than traditional models in predicting the total wind energy generated by wind farms worldwide.
Google has made its GenCast model open-source, releasing its code, weights, and forecasts. This move is intended to facilitate collaboration with academic researchers, meteorologists, data scientists, and other organisations in areas like disaster response and energy planning. By making the model available to a broader community, the goal is to support further advancements in weather forecasting technology. The company also plans to release real-time and historical forecasts generated by GenCast, enabling users to integrate these insights into their own research or applications.
In addition to GenCast, other tech giants are advancing AI models for weather and climate prediction. Earlier this year, Nvidia Research introduced StormCast, a generative AI model aimed at simulating high-fidelity atmospheric dynamics. Similarly, IBM announced an AI foundation model to address various weather and climate challenges. It was developed in collaboration with NASA and with support from Oak Ridge National Laboratory.