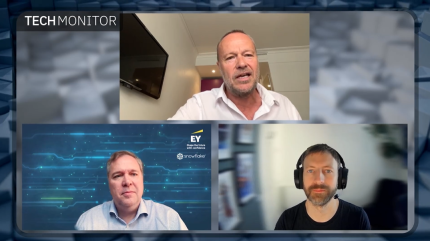
The financial services sector has long been an early adopter of automation and AI technologies. However, not all firms are seeing the ROI they expected from GenAI. Where, therefore, must these companies develop new skills and strategies? And, from data privacy and security concerns to the ethical implications of AI-driven decision-making, what risks and roadblocks still need to be overcome? To address these questions and more, Tech Monitor associate editor Jon Bernstein was joined by Frank Chevalier, EY EMEIA Financial Services AI & Data Leader at EY, and Michael Taylor, Field CTO, AI/ML, Snowflake, for the latest instalment in our Power of the Possible series, titled: “How might generative AI transform financial services?”
The human factor
While that transformation may still be some way from realisation, momentum is gathering pace. 77% of leaders questioned for EY’s most recent European Financial Services AI Survey expected GenAI to significantly affect productivity, with 68% believing up to a quarter of all roles required imminent upskilling. Despite this, over a third still lacked concrete action plans.
“It comes down to the enhancement of human capabilities,” Chevalier said in response to where he was seeing most Banking, Financial Services, and Insurance (“BFSI”) investment in GenAI. “It’s a symbiosis, where the tech drives substantial improvements in efficiency and decision making.”
While the sector has long been at the forefront of using new digital and data tools to enhance performance, for Taylor, this new stage represents more than merely a step change. “Yes, there’s a track record of using numerical methods in financial services, but a lot of that has been tapped out – there’s only so much extra alpha you can squeeze out in an already efficient market,” he explained. “But where you will be seeing real impact is in putting knowledge at the fingertips of high cost, high skill resources. That human-in-the-loop approach also means you can more easily smooth off the rough edges that are somewhat inevitable with any new technology.”
Navigating the regulatory landscape
But the challenges for BFSI go far beyond rough edges. As Taylor was keen to point out, arguably only healthcare deals in more sensitive data sets, with any misstep having huge potential consequences. “The move fast and break things philosophy found in tech is not something financial services has the benefit of leaning into,” he observed. Staying on the right side of regulation while still fostering innovation is the key here.”
Managing this requires getting the right human and tech elements in place, which can bring considerable changes in how one stores and manages data. “Transitioning to a cloud-based solution is imperative,” Chevalier said of leveraging GenAI effectively. “For proof of concept and proof of value, there is a tendency to use traditional or on-premise tools, but it requires a really robust talent pool and advanced capabilities to be able to sustain that into the long term. Being able to manage high-quality, accessible data, and integrating generic large language models with proprietary data, for example, is a critical challenge.”
The number of nuanced, complex decisions that must be made around what data is relevant, legal, and applicable means retaining human input is essential. The picture is further complicated both by a fast-evolving regulatory landscape, and the fact that so much of BFSI activity is cross-regional. Indeed, for Taylor, regulatory complexity should play a significant role in informing where enterprises begin their GenAI initiatives.
“Pick something that isn’t under the same scrutiny,” he advised, citing the example of a relationship manager providing the house view on a particular set of investments. “The house view already exists in some fashion, so that is data that can be harvested and it is information that the bank was already going to share, so there are no privacy issues. Indeed, it may have already been shared through various means such as mailshots, but you now have a solution identifying and pulling all of that in.
“The regulatory stakes are low, so the focus can all be on getting the LM, the hosting and the UX right. Establishing that robust implementation and strategy, and the confidence it brings, means you can then move onto slightly higher-risk areas, where the delivery portion is already set and you can fully focus on the regulatory aspect.”
Simplifying the complex
Chevalier cited an example of an initiative EY had helped implement for a European bank, transforming official communications from rating agencies and analysts into actionable advisory materials, including videos, for clients. “You see AI not only streamlining information dissemination but also adding value by making complex data much more accessible,” he explained. “It also addresses a new generation who want to be served information in a new way, converting static text into live material.”
Bringing such business value through streamlining and simplification lies at the very heart of what financial enterprises should be aiming towards. It was an overarching message from both interviewees that the empowerment of one’s own knowledge and talent bases remain a driving factor behind GenAI integration.
“Make your life easy,” Taylor advised. “Use SaaS solutions. Use consultancies. Do whatever it takes to remove complexity from your solution and have your team focus on the activities where they are most differentiated.”
To learn more, download Snowflake’s AI Blueprint for Financial Services here. The opinions in this article are those of the author and do not necessarily reflect the views of the global EY organisation or its member firms.