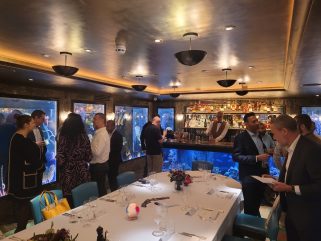
While the aims of digital transformation remain unchanged – to drive productivity, foster innovation, and offer a competitive edge – the means to achieve it are changing. Artificial intelligence and generative AI (GenAI), in particular, are opening up new opportunities. New use cases abound.
To underscore the power and potential of GenAI, organisations need to review their infrastructure to ensure that it is fit for purpose. Can it handle AI workloads? Does it have the scale and elasticity to manage a step change in data processing? Will it protect the integrity of your intellectual property? Will it keep your network and applications safe? And your customer and employee data secure?
These interconnected issues – at the heart of digital transformation in late 2024 – formed the basis of an October Tech Monitor discussion. The roundtable event, in association with Cisco, explored what organisations across the economy require to get ready for the next wave of transformation.
AI use cases
The evening began with a collection of AI use cases – those that attendees are contemplating, testing as a proof of concept (PoC), or deploying. Most examples focused on efficiency savings. One organisation represented around the table is testing AI to help it streamline its support ticketing process. Issues raised by users often involve several dozen touchpoints before resolution. By assimilating a mixture of structured and unstructured data sources, the AI application can identify correlations and create standard approaches to the problems that arise persistently.
Another is using AI to help manage its procurement processes. By tagging, classifying and consolidating sources, the organisation is able to automate manual tasks and address the majority of cases that don’t get handled today by an overstretched team.
There were plenty of examples elsewhere of productivity-focused applications of AI – from an app that creates briefing notes for executives ahead of important meetings to tools that generate meeting notes and minutes.
Augmentation of human code writing is another common and popular use of AI. It was an appropriate topic, too. On the day of the event, Google announced that it now uses AI systems, overseen by human programmers, to generate more than 25% of new code for its products.
Implementation and buy-in
There was a degree of disagreement about the best strategic approach to get support from senior leadership. For some, small-scale projects that demonstrate efficiency savings are the best place to start. These are likely to be more manageable and quicker to implement. By demonstrating – albeit on a relatively small scale – the potential of AI, those in an organisation wishing to champion the bigger possibility of the technology, gain credibility and, subsequently, buy-in for their future ambitions.
As a counterpoint, another attendee argued that incremental adoption of AI – such as features fused onto existing applications – doesn’t cut it. She described these as “non-events” that fail to gain traction with leadership teams who are more likely to “shrug their shoulders”. To truly get the attention of senior decision-makers, “big bang” ideas are required.
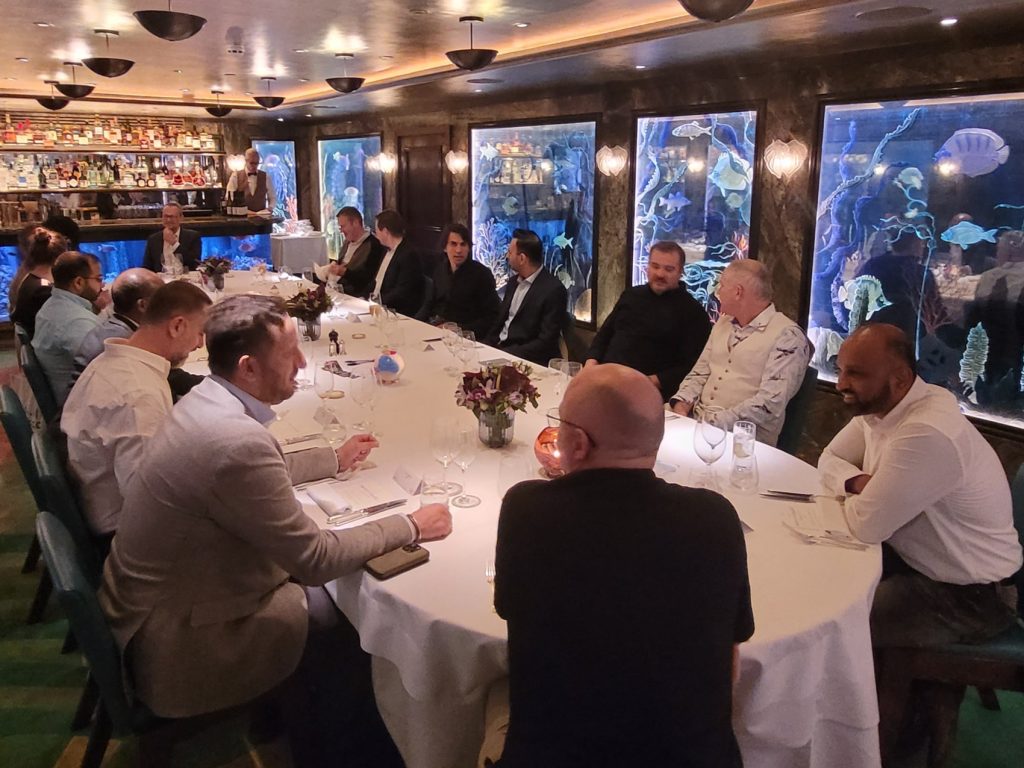
Infrastructure needs
Next the discussion turned to infrastructure and whether, if at all, the networking, computing, and software toolkits that underpin high-consumption workloads are a consideration when organisations embark on AI projects. Across the tech stack, do those championing AI appreciate the infrastructure demands involved? A straw poll of attendees suggests that some do, and some don’t.
One firm represented around the table understood the limits of its infrastructure. In other words, it knew it could undertake a PoC based on two months’ worth of data. However, to deploy a full-scale application, requiring 12 months of data or more, would necessitate bolstered infrastructure. Another attendee anticipated that given the major manufacturing-style workloads already in operation, existing infrastructure would be able to accommodate AI-based use cases. “Don’t be so sure,” this attendee was advised, a nod to the compute-hungry nature of large language model-based services.
Some organisations, meanwhile, outsource part of the infrastructure overhead by operating AI workloads in the cloud or by relying solely on SaaS (software-as-a-service) applications.
Infrastructure costs
Elsewhere, the talk turned to cost. To help anticipate and manage future needs, one attendee made a plea for some form of metering, or an ‘all-you-can-eat’ system of infrastructure procurement. Having little visibility on what your infrastructure bill might be month-on-month makes any return on investment projection impossible, this attendee pointed out.
The viability of projects – and AI projects in particular – rests on knowing what the underlying networking, hardware, and software are going to cost. “If you could lock in the infrastructure costs over a period of time, that would be very attractive,” the attendee said.
A view from Cisco: Pick an AI use case
Post-event, Cristi Grigore, Sales Engineering Manager, UK Enterprise at Cisco, offered his verdict on the evening’s discussion.
“There is clearly a great deal of interest in how to use AI, especially in making processes more cost-effective and efficient,” he told Tech Monitor. “However, we are still in the proving stage. There are a lot of PoCs but we have yet to identify the killer use cases in most organisations.
“If you think of it in terms of the Gartner technology hype cycle that means we haven’t reached the top yet and at some point, we are going to head to the ‘trough of disillusionment’. There are going to be questions. So my recommendation is to pick a use case and prove it properly. Don’t try and boil the ocean. Identify a discrete use case, focus on it, and execute expertly to prove the value.”
AI infrastructure: time to consider the impact
On the infrastructure required to support AI workloads, he said: “Most people aren’t thinking about how they could deliver their use cases at scale and, therefore, the impact and requirements that would have on their infrastructure.”
Grigore suggested that there is a “disconnect” between the “technology-inclined” and “business-focused”. The latter, he said, are more likely to leverage cloud, effectively outsourcing infrastructure needs in the short term. The former is more aware, he said, of requirements “as you move down the technology stack.”
AI infrastructure: making the cost case
Finally, he acknowledged – as suggested during the roundtable – that there is a desire for more certainty around the cost of infrastructure. “You do need to link costs with benefits and it can be difficult to find out what the costs are,” Grigore told Tech Monitor.
“This isn’t an AI-only problem. This is a wider problem for any application use case. There are tools and methods that provide more visibility on the cost. It is also about the culture of the organisation – how they are set up from a financial perspective, where the budgets lie, and how complex the environment is. It is easy to see where the application is running if you have the right visibility and telemetry – and then it’s a case of having the right tools to assign the right budget in the right place.”
Cisco, he said, can support organisations to understand the true cost of projects. He pointed to Cisco AI PODs which are designed for plug-and-play deployment, helping organisations to integrate projects into existing data centres or cloud investment.
‘Preparing for digital transformation in the age of AI’ – a Tech Monitor executive roundtable event in association with Cisco – took place on Wednesday 30 October at Scott’s Mayfair Restaurant, London.