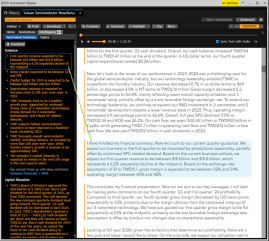
Pity the investment analysts. Tasked with trawling through vast amounts of esoteric information on everything from balance sheets to earnings calls before building fiendishly complex models to justify buying or selling a given asset, these financial gumshoes are the unsung heroes of the banking world – unsung, one suspects, because the glory of the final decision on when to buy or sell those assets is ultimately claimed higher up the corporate food chain.
At least one company is betting, however, that the industry’s rapacious appetite for restructuring extends to the responsibilities of its analyst class. Bloomberg’s new ‘AI-Powered Earnings Call Summaries’ promise to feed that beast by trawling through vast amounts of publicly available data on corporates before spitting out analyses rivalling its sweaty human antecedents. Indeed, Bloomberg terminal users can now access AI-authored summaries and analyses of company performance to unearth valuable insights and, potentially, steal a march on their competitors.
These tools, explains Amanda Stent, are the fruit of a decade-long project of internal AI development – and, she is keen to add, designed to assist rather than eliminate the humble analysts.
“Generative AI is useful for analysts who spend days trying to find and synthesise relevant information,” says Bloomberg’s head of AI strategy and research. “It makes that process easier, especially with unstructured documents. Finding information on a company and comparing it with others normally involves researching each competitor.”
In other words, there’s that bit less drudgery in the daily life of your average banking gumshoe. “With Gen AI, you just ask the right question,” says Stent. “It frees up time to add your special sauce as an analyst.”
Insight and efficiency
Executing this automation hasn’t been easy, Stent stresses. “Many people think you can just lay AI on top like icing on a cake,” she says, “but to make it work you must reorganise all your data and redo your technology infrastructure.”
Part of this effort involves the training of large language models (LLMs) in the cloud. Using real historical data, models can learn to understand how to derive meaningful insights, and Bloomberg has been working closely with AWS on these training initiatives.
Bloomberg also recently announced a collaboration with application networking and security specialists Tetrate on a new Cloud Native Computing Foundation (CNCF) community open-source project – the ‘Envoy AI Gateway’ – which will provide key infrastructure that will sit in front of multiple LLMs to handle authentication, rate limiting, and other features for enterprise teams building and managing generative AI applications.
Bloomberg’s system has learnt from the best, having been trained by hundreds of the company’s analysts to generate relevant information –including summaries of earnings calls with embedded links to source documents.
“We get humans to do the tagging to identify the topic, for example, and to step in when the model is running if the system is unsure or a problem arises,” Stent explains. “We also get humans to check summaries to update and train our models. There is constant management because AI products are always evolving.”
The latest tools mark an evolution in how the investment industry uses AI. Its power to rapidly and accurately process large volumes of data gives it a natural home in the data-heavy investment sector, but its adoption has been slowed by a degree of scepticism that is natural in the heavily regulated financial services industry.
Initially, a major concern revolved around the so-called ‘black box’ nature of certain LLMs – namely, that the chain of reasoning behind certain decisions could be so opaque as to be completely unknowable, raising clear issues about accuracy and, ultimately, accountability in the use of such products. Both are considered to be a sine qua none across the financial services industryy; hence the sector’s cautious enthusiasm for ‘explainable AI’, where analysts and investors can see how advice was generated by a given model. As such, outputs are heavily footnoted, so that a regulator or client can see how a particular recommendation was reached. This is vital when people’s money – perhaps their life savings – is at stake.
Widespread acceptance and trust will take some time to build. To help achieve that, Bloomberg leverages not only its vast data organisation, but also its experts to ensure that data is enriched, accurately tagged, and that there is sufficient human oversight to ensure transparency.
Stent highlights that there are both opportunities and challenges in deploying AI in the investment arena – hence the constant monitoring. Vijay Raghavan, senior analyst at Forrester, is also keenly aware of both. “Earnings calls are scripted and happen regularly, so the adoption of an AI summary tool should have a high take-up rate,” he remarks. “It comes from data, documents, and a written transcript that must be submitted to regulators, so summaries are straightforward.”
Bloomberg’s toolkit can certainly drive efficiencies and provide initial insights, but analysts cannot hand over full responsibility for the output. They will still need to add their own original qualitative analysis about what different outputs from the data represent and how they connect to create a narrative. “The potential pitfall lies in simply taking the output as truth,” he adds. “AI cannot spot tone of voice in calls, or understand metaphor or irony. It just picks up the transcripts.”
Raghavan agrees that summarising themes is a great starting point for analysts, but feels it is still incumbent on them to check the outputs and come to their own conclusions. “The danger is that everyone is going to have the same opinion,” he says. “So, they can use it as an efficiency tool for quick summaries, but analysts still have to contribute their own thoughts.”
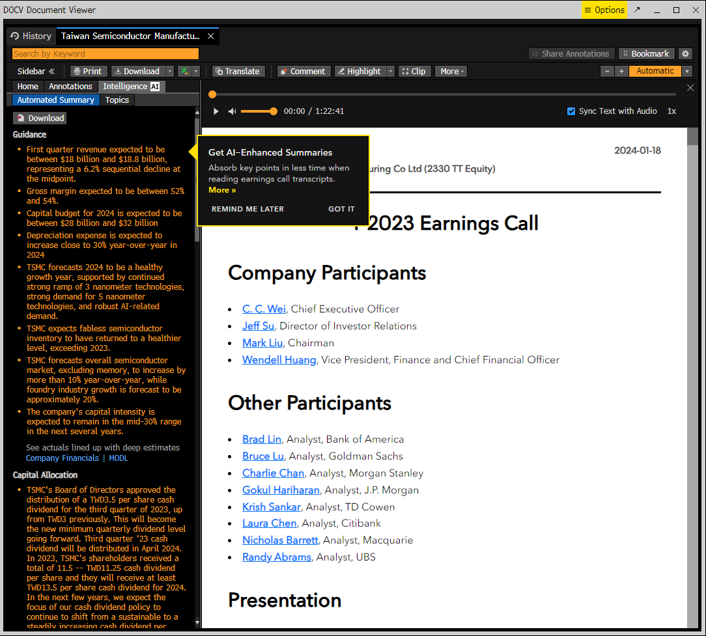
The real power of AI-powered earnings call summaries
Raghavan also points out a key factor in deriving real value from Gen AI – figuring out the right questions that will elicit factual, complete and relevant information. Specificity is key, so analysts must think carefully about prompts.
“With generative AI you need the right questions and prompts before you even get close to what you are looking for,” he says. “More nuance is needed with this kind of AI, and you have to be patient and rephrase questions sometimes. Financial advisors will need more training to understand how to interact with it effectively.”
With time and education, the value of Gen AI will undoubtedly grow, but its progress must be steady. “Every firm is experimenting with this technology, but in a heavily regulated industry they are being cautious,” says Raghavan. “It is moving slowly from the back office towards the front office, and there will always be a human in the loop to ensure the outputs make sense.”
As trust grows, generative AI will carve out a bigger niche in the toolkit for investment advisors, and Bloomberg’s product seems to be a big step forward. As we approach 2025, AI is becoming ‘ready for prime time’, as Raghavan puts it – but the guardrails are still firmly on.
“The big advantage is that it saves time, freeing up people for value-added services, but eventually AI will make its way right in front of customers,” says the analyst, but cautions advocates to temper their enthusiasm. “Our expectations of what it can do right now,” argues Raghavan, “are too high.”