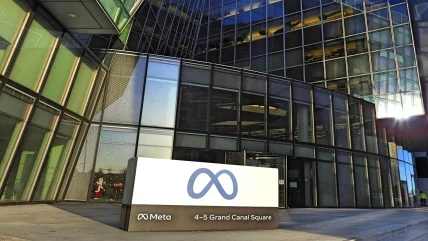
Meta has unveiled several new artificial intelligence (AI) models, including one designed to evaluate other AI models, as part of its ongoing efforts to advance advanced machine intelligence (AMI). These models are aimed at enhancing AI capabilities across multiple domains, continuing Meta’s focus on fostering innovation and collaboration within the AI research community.
The newly released models include the Self-Taught Evaluator model, Segment Anything Model (SAM) 2.1, Meta Spirit LM, Layer Skip, SALSA, and Meta Lingua, among others. Each of these models focuses on different aspects of AI, such as perception, speech, language, reasoning, and alignment.
The Self-Taught Evaluator is a model designed to train reward systems using synthetic data, eliminating the need for human annotations. By using a so-called ‘LLM-as-a-Judge’ mechanism, the Self-Taught Evaluator is designed to iteratively enhance the performance of other models, leading to significant efficiency gains on platforms like RewardBench. Meta claims that the model represents a step forward in reward modelling, enabling more scalable and rapid training processes.
The updated SAM 2.1 incorporates data augmentation techniques and enhanced occlusion handling. SAM 2.1 is widely used in fields such as medical imaging and meteorology for its ability to segment smaller and more visually similar objects.
Meta has also released the SAM 2 Developer Suite, which includes open-source code for researchers and developers interested in customising and fine-tuning the model. Meta Spirit LM, meanwhile, is a multimodal language model that integrates both text and speech using a word-level interleaving technique. This model comes in two distinct versions: Spirit LM Base, which utilises phonetic tokens, and Spirit LM Expressive, which uses pitch and style tokens to capture tone, including emotions like excitement or anger.
These models support tasks that include automatic speech recognition, text-to-speech conversion, and speech classification, providing more natural and contextually rich outputs.
Layer Skip is a model focused on optimising the performance of large language models (LLMs) by executing selective layers and verifying outputs through subsequent layers. This approach reduces computational costs, improving both energy efficiency and performance.
Additionally, Meta has released fine-tuned checkpoints for models such as Llama 3, which demonstrate improved efficiency and accuracy with early exits. The Layer Skip technique is expected to contribute to ongoing research in AI optimisation by providing an energy-efficient pathway for deploying large models.
For its part, the SALSA model focuses on benchmarking AI-based attacks against lattice-based cryptography, specifically targeting sparse secrets in these cryptographic standards. Lattice-based cryptography, recommended by the National Institute of Standards and Technology (NIST), is a foundational element of post-quantum cryptography (PQC). SALSA’s capabilities are designed to help validate and improve the security of these systems, ensuring resilience against potential AI-driven threats.
Meta Lingua, is a lightweight, modular codebase for training language models at scale. It aims to reduce the technical complexities involved in model training, allowing researchers to focus on experimental design rather than infrastructural hurdles. By simplifying the model training process, Meta Lingua supports rapid experimentation and facilitates the practical translation of conceptual AI ideas into tangible results.
Meta continues to court the open-source AI developer community
AI experts have argued that Meta’s new models are limited in several key respects. Critics allege, for example, that SAM 2.1 still struggles with complex segmentation in medical and meteorological tasks, while Layer Skip’s efficiency could ironically compromise its precision, limiting use in finance and law. The Self-Taught Evaluator’s reliance on synthetic data, meanwhile, raises bias concerns in healthcare, and SALSA’s scalability remains questionable beyond specific cryptographic standards.
Others in the open-source community have criticised Meta for labelling their models ‘open-source’ when, in reality, most aspects of their operation remain private. According to the Open Source Initiative’s chief Stefano Maffulli, the social media giant was “polluting” the term, telling the Financial Times that the firm’s use of the term was “damaging” at a time when jurisdictions like the EU were seeking to champion innovation in the sector.