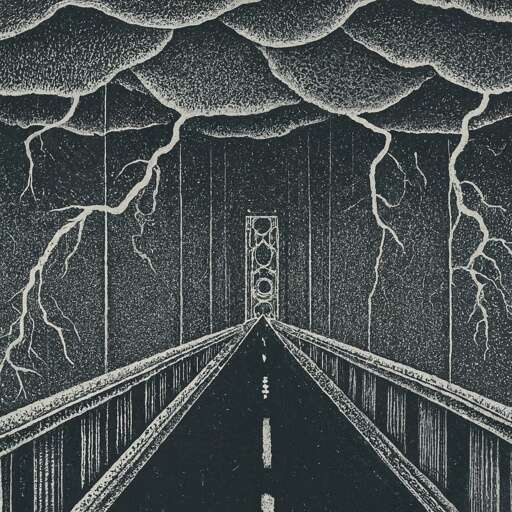
The days are over when extreme weather conditions, such as devastating storms, floods, heatwaves and droughts, were considered “once-in-a-century” occurrences. We need only look so far as recent events in the UK, where weather experts have warned of what will likely be the stormiest winter since records began, to know that this is true.
As the climate crisis worsens, these events will only multiply – as will disruption to critical national infrastructure. In many instances, these are faults and weaknesses that might have been spotted or fixed during the next round of scheduled maintenance. When extreme weather strikes, however, it is too late to fix things, and the costs – both in terms of money and human impact – can escalate rapidly.
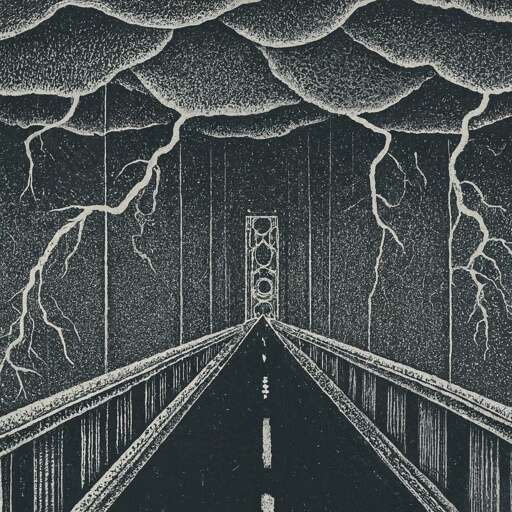
Enter predictive maintenance. Like our scarily accurate weather forecasts, predictive maintenance systems use AI to crunch data from sensors atop drones, satellites and cameras to make calculated bets on when, where and how infrastructure might fail under the stress of extreme weather. Using long-established techniques like computer vision, image analytics, deep learning and basic pattern recognition, these applications can alert maintenance teams to anomalies or problems that humans wouldn’t be able to spot themselves without assistance.
Thanks to predictive maintenance, organisations can dramatically boost the availability of their assets, increasing equipment uptime by 10% to 20% and reducing downtime by up to 50% – which, by conservative estimates, reduces overall maintenance costs by between 12% and 18%. Depending on the use case, predictive maintenance can also reduce the overall cost of infrastructure upkeep in the short- and medium-term by between 10% and 40%. These percentages translate into massive real-life savings. After all, unplanned downtime costs manufacturers an estimated $50 billion every year – nearly half of which is caused by equipment failures.
Predictive maintenance involves translating knowledge into action
The key challenge for companies lies in translating the incoming data into their operations. Simply connecting sensors to an IoT platform alone will not help a company to make sense of messy real-world problems, especially during times of crisis. That’s why predictive maintenance solutions must pay attention to an asset’s entire solution lifecycle – from concept and implementation to measuring business outcomes long after the platform has been installed. Consideration of weak points that could potentially fail during extreme weather has to be one of the business drivers for a predictive maintenance programme.
In the grand cost/benefit analysis, such an approach may not deliver an immediate return on investment – but this approach to predictive maintenance can help to fix weaknesses before extreme weather conditions result in catastrophic failures. That’s especially true now, as qualitative leaps in generative AI mean that predictive maintenance systems can alert organisations in natural language to immediate problems. From there, maintenance teams can also query the system using conversational prompts.
Adaptation and mitigation have become two of the key objectives as our world readies for the impact of climate change. The growing shift towards technology-driven solutions is crucial for ensuring safety and reducing economic losses in an era of increasingly unpredictable climate patterns. As such, predictive maintenance will play a vital role in supporting our objectives as we work towards making our world more resilient.