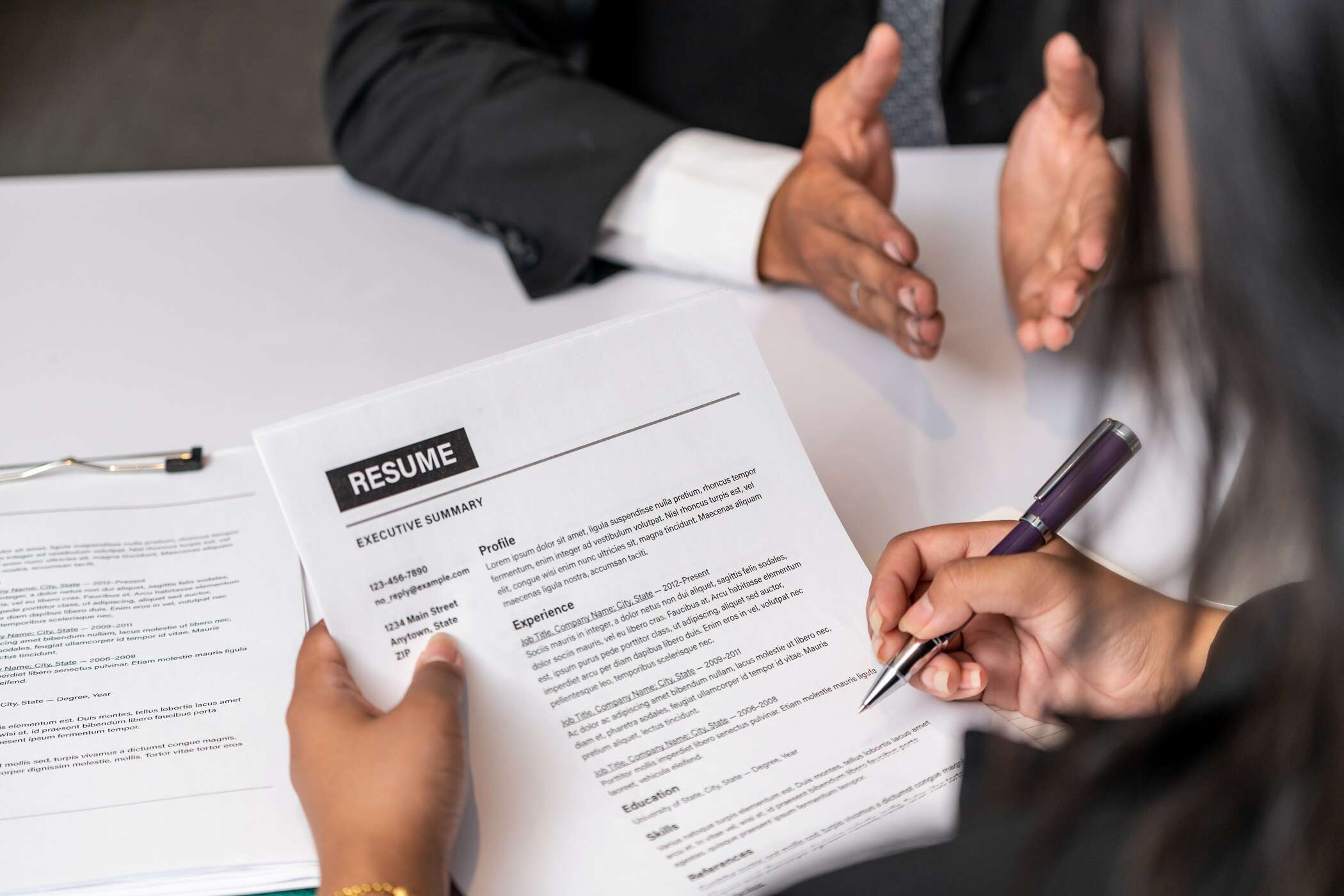
Automated recruitment software is erroneously discarding millions of job applications from qualified candidates, according to new research. These so-called ‘hidden workers’ are often rejected because they don’t fit into predefined categories, according to the Harvard Business School study.
The study, Hidden Workers: Untapped Talent, finds that “long-standing and widespread management practices contribute significantly to constraining the candidates that companies will consider, leading to the creation of a diverse population of aspiring workers who are screened out of consideration – or ‘hidden’.”
Digital tools such as applicant tracking systems (ATS), which help manage recruitment workflows, and recruiting management or marketing systems (RMS), which are often used to advertise positions, are a key cause of this problem, the authors say. But with such tools now embedded in the recruitment process – more than 90% of businesses polled in the study use an RMS to filter or rank candidates – attention is turning to how they can be used more fairly.
At a time when the technology sector and many other industries face skills shortages, better auditing and regulation of recruitment software could help root out potential discrimination and bias.
What are ‘hidden workers’ and why are they hidden?
The study defines hidden workers as those who want to work but can’t find suitable employment due to their personal circumstances disadvantaging them in the hiring processes. “They experience distress and discouragement when their regular efforts to seek employment consistently fail,” it says. The authors, who spoke to 8,000 hidden workers and more than 2,250 executives across the US, UK and Germany as part of their research, estimate the number of hidden workers in the US alone is 27 million.
While other factors, such as insufficient training, lead to these people being excluded from recruitment processes, automated systems are making their lives even harder. They are "designed to maximise the efficiency of the process," the report says. "That leads them to hone in on candidates, using very specific parameters, in order to minimise the number of applicants that are actively considered."
Joseph Miller, one of the study's authors, said in an interview with the Wall Street Journal that one example of this came from a hospital looking for workers to input patient data, which only accepted candidates with "computer programming" on their CV.
The findings of the study come as no surprise to Anna Thomas, director of the Institute for the Future of Work think tank. "There is huge potential to broaden the talent pool with these kind of tools but in reality, they're not used in that way," she says. "People think automated tools are inherently neutral, but they are trained on datasets which reflect existing inequalities, so unless you investigate and correct these inequalities they get projected into the future."
Thomas says the use of automated hiring systems has increased dramatically during the Covid-19 pandemic, particularly for companies looking to hire lots of extra staff rapidly. She uses the example of Amazon, which reportedly hired 1,400 workers a day last year as demand for its services increased exponentially. But, she says, for many companies, automated hiring has been introduced "extremely fast and not done in a thoughtful way, without a full understanding of what the impacts might be in the medium or long term".
How to solve the problems with AI recruitment
The Harvard Business School study's recommendations for improving the chances of hidden workers landing the roles they seek include the suggestion of a fundamental change in the way ATS and RMS software works. At present, it focuses on "negative" filters – excluding applications without required information - but the authors believe a fairer solution would be to apply an "affirmative" logic instead, highlighting applications with some, if not all, that meet some of the requirements. This would seem "a more logical approach for seeking talent," it says, adding: "Configuring systems to identify applicants with the specific skills and experiences associated with fulfilling the core requirements of the role would promise to be more efficient and inclusive."
It also recommends rethinking the application process "through a user experience lens", as 84% of hidden workers surveyed said they find this initial part of the process difficult. A redesigned application could "ensure that the skills and credentials requirements are accessible at the beginning of the process and that the timetable and criteria for decision making is clear," the report says.
Thomas believes better regulation is key to avoiding problems with automated recruitment software. "It needs to be regulated properly, and we need to move towards pre-emptive compulsory evaluation of algorithmic impacts," she says. "There also needs to be rigorous ongoing algorithmic impact assessment that looks at impacts on workers and people, the trade-offs that are being made and any adjustments needed to mitigate potential inequalities."
Whether policymakers in Europe or the UK will address this issue remains to be seen, with the UK government currently drafting its post-Brexit AI strategy. Thomas says the EU's proposed AI regulations "aren't actually very rigorous or thorough" on this topic. "It doesn't really deal with the risks and potential harms identified in the workplace," she says. "So there is space for the UK to do better if there's an appetite to do so."
Home page photo by Blue Planet Studio/Shutterstock