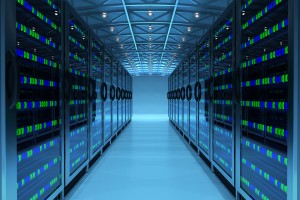
Making big data sets actionable is a perennial challenge for businesses. With application and infrastructure workloads increasingly spanning multi-cloud architectures and IT silos, while also needing to keep pace with blisteringly paced technology cycles, the challenge is increasingly beyond the ability of human cognition.
For Virginia-headquartered IT services specialist ScienceLogic, the answer is a formidable step up in machine learning (ML) and after three years of development and numerous patents, the company this afternoon (13:00 GMT) is launching a new suite of AIOps tools, dubbed “SL1” that are intended to level the playing field between the speed at which Dev teams create services, and Ops teams can deploy and maintain them.
Computer Business Review pulled CTO Antonio Piraino aside to find out why these tools, are “capable of supporting Russian instrumentation on the ISS and sugar dispensers on Kellogg cereal lines” and what the game plan is.
What powers your data discovery tech?
We’ve today unveiling two core technologies – PowerSync and PowerMap.
PowerSync allows for automated discovery and data synchronization using light-weight agent and agentless technologies that reach diverse endpoints irrespective of their nature. We have successfully discovered over 5,000 unique device signatures with nearly 200 APIs to establish a 12x advantage over our nearest competitor.
PowerSync is so powerful that it is capable of supporting Russian instrumentation on the ISS, sugar dispensers on Kellogg cereal lines or KonicaMinolta’s Edge Computing platforms in their Digital Hub offering. With this unmatched discovery capability, we are able to build an operational data lake in real-time and use PowerSync to push out data on demand to IT ecosystem partners to enrich and automate their platforms.
PowerMaps is our secret sauce, where we bring context to the data feeding advanced Neural Nets with training data information built on topology maps. Once we see all the data, we are able to build dependency maps between applications and their underlying infrastructure in real-time. These maps are critical to know how to identify root-cause analysis and help fix performance issues at machine speed.
Everyone is launching ML/AI capabilities to make sense of data. What makes your offering stand out?
There are two aspects of AI/ML, with much focus on the smart algorithms that determine patterns and predictive insights. Our focus is on providing training data to feed an IBM Watson-like AI engine. As Jeff Dean of Google AI has stated, it’s the training data that determines quality results of the most sophisticated AI engines and not the algorithms themselves – garbage in, garbage out.
ScienceLogic with SL1 is focused on building the most comprehensive operational data lake in real-time with context through topology maps. Our partnership with IBM and Watson is a great example of how we are driving predictive insights that, in turn, drive self-learning AIOps systems, resulting in predictive insights for enterprises.
How do you envision SL1 being used by industry?
Our work with IBM and Watson is to help enterprises move at machine speed, to keep pace with ephemeral digital platforms. Building a great digital experience is only as good as a resilient digital platform. DevOps movements prize speed and agility and yet desire resiliency. This is what we hope to bring by using Watson and predictive insights to identify anomalies in mission-critical business services and remediate before they impact the consumer experience.
KonicaMinolta is another great example of an enterprise that’s undergoing business model transformation, shifting from hardware to IT services. Konica has deployed their edge computing and IoT platform with the Workplace Hub. SL1 can analyze and connect millions of metrics per second, enabled by a horizontally scalable NoSQL architecture and a topology-based analytics engine. This enables automated root cause analysis, proactive remediation and smart provisioning to millions of edge devices via Konica’s AI-enabled management platform.
You mention “Automated topology maps to establish real-time relationships between disparate data sets bringing context to data”. What does this mean, in plain English?
These topology maps are core to how we bring context to the training data for AIOps. Data without context equals noise; 71 percent of enterprises today say their big data strategies are not actionable. Major AI platforms spend overwhelming compute cycles to determine application to infrastructure relationships, traversing private and public clouds in order to determine patterns and drive automation in how they fix business-impacting issues. We at ScienceLogic can with 100 percent accuracy provide those application to infrastructure relationships so that AI engines like IBM Watson optimize their use of compute cycles and, more importantly, improve accuracy of their analysis and drive automation towards resolution with confidence.
Inaccurate topology can be worse than no topology at all since it can lead to a false sense of security that the impacts of a problem are all understood and are being dealt with. The reality of modern application deployment architectures is that topologies continuously change through a myriad of mechanisms from vMotion to Elastic Load Balancing to ephemeral containers. With PowerMaps these topology changes are immediately captured from across the complete technology stack and used to enable a complete and accurate dependency map supporting impact and root cause analysis.
See also: AWS Machine Learning Use up 250%