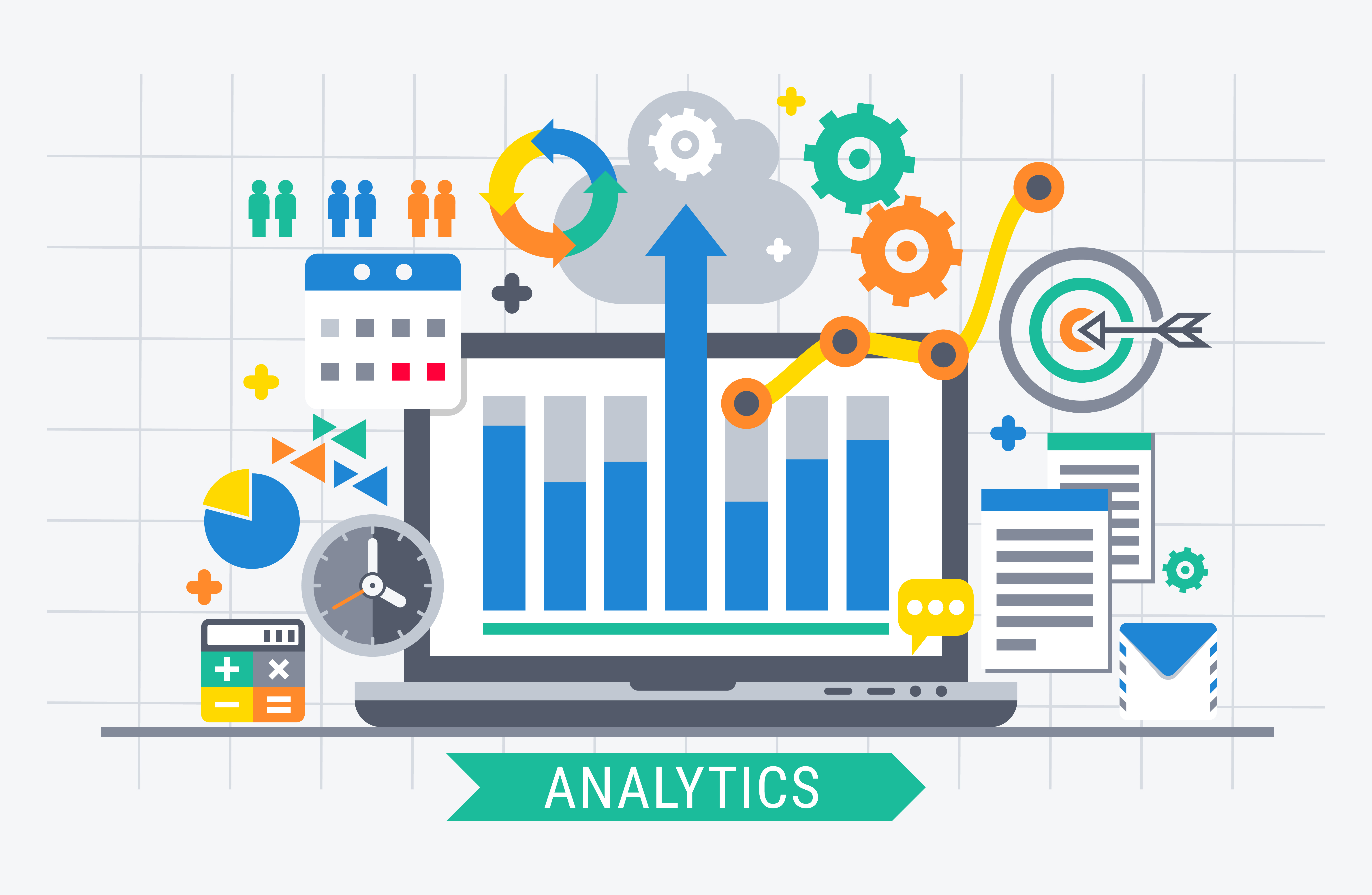
EB: What is predictive analytics and what is the value of such analytics to businesses today?
AB: Predictive analytics involves using data, statistical algorithms and machine learning techniques to predict the likelihood of future trends and business results, based on past trends and data.

It brings together a number of different advanced analytics disciplines and technologies, from statistical analysis and deep data mining through to predictive modelling and machine learning, predicting the most likely future outcomes and answering valuable questions for organisations such as, “what will happen?” or “how are customers likely to react to this?”
Predictive analytics is about producing valuable business insights and accurate foresights or forecasts from large sets of data, to help users decide on the next-best course of action and provide better customer experiences.
The increasing volumes of data available, combined with faster, cheaper computing power, and the development of more user-friendly analytics and AI software; like Salesforce Einstein, have enticed an increasing number of companies to leverage predictive analytics to achieve their business goals and gain a competitive advantage.
75% of companies increasing investment in analytics cite revenue gains
The value of predictive analytics is clear. It makes sense of big data, it helps to inform decision-makers and it gives them “actionable insights”.
According to Bluewolf’s most recent State of Salesforce report, based on a survey of more than 1,700 Salesforce customers, 75% of companies increasing investment in analytics can cite revenue gains as a measurable business outcome.
Most interestingly, the report revealed that 81% of Salesforce customers cited increasing the use of predictive analytics as the most important initiative for their sales strategies.
So what is predictive analytics? Put as simply as possible, there are four levels of analytics, from descriptive analytics, which describes the real world in concrete data, to diagnostic analytics, which looks at historical data to provide insights as to why things happened as they did in the past, through to predictive and prescriptive analytics.
It is these latter two areas of advanced analytics, where processes are increasingly automated, that are of immense value to businesses looking to make sense of big data.
Organisations can confidently look forward and anticipate different business outcomes based on predictive analytics, and not merely on a hunch or a gut-feeling. Prescriptive analytics takes automation, and augmentation, of the decision-making process a step further, offering the business answers to questions such as “how should we respond to X?” “Or what do we need to do now to make Y occur?”
EB: How does machine learning and AI factor into predictive analytics?
AB: Artificial Intelligence (AI) and machine learning are the next step of analytics. Where cognitive computing systems constantly learn about your business and intelligently predict industry trends, customers’ needs and more.
One of the key findings of the Bluewolf State of Salesforce report is that increasing investments in making analytics actionable and accessible to staff and customers is the best way to move applications from just smart, to intelligent.
Beyond this, while half of companies surveyed consider their core applications to be intelligent, few companies have yet to reach the level of cognitive applications, which are defined by the following four characteristics.
Cognitive applications:
Understand unstructured data, like language and imagery, just as humans do
They can reason, infer, and extract ideas
With each interaction, they sharpen their expertise so they never stop learning
With abilities to see, talk, and hear, they interact with humans in a more natural way
AI and machine learning help businesses uncover patterns of relationships between hundreds of different factors, relationships, causes, interactions and non-linear systems in order to understand how certain outcomes have been reached.
With intelligent and cognitive applications enabling the business to replicate the routes to outcomes in order to improve the brand experience to that most desired by the customer.
EB: What would be your top tips to a business looking to deploy predictive analytics?
AB: My five key thoughts for businesses looking to deploy predictive analytics are:
Garbage In, Garbage Out:
Predictive analytics is only as accurate as the quality of the original sets of data sets mined. Ensure that you are constantly honing your analytics processes to get the most valuable insights and predictions for the future from the data you have.
Understand the Business Problem:

You need to understand both the data being used for a predictive analysis exercise and the business problems being addressed.
Find The Best Tools:
Once you understand the data and business problem find the right software and the right expertise to build and refine the predictive models.
Nobody Has Perfect Data:
There is a misconception that pristine data is a pre-requisite for using predictive data tools. Not so. No business has perfect data.
Iteration Is Key:
However sophisticated your raw data, predictive analytics offers a competitive edge. Start with what you have and improve your data set as you go.
Start now by applying predictive analytics to the data you hold, reinvest the benefits into improving data and systems, and you are firmly on the path to deploying AI to gain truly intelligent augmentation for your business decision making, delighting customers by anticipating their needs and seizing a competitive advantage over your peers.